Efficient representations of tumor diversity with paired DNA-RNA aberrations
Efficient representations of tumor diversity with paired DNA-RNA aberrations
Cancer cells exhibit significant dysregulation in key regulatory pathways due to well-documented mutations and other DNA-related abnormalities. These disruptions, however, are not uniform; they display considerable heterogeneity in their identity, frequency, and location across individuals with the same cancer type or subtype. This variation naturally extends to the transcriptome, resulting in a wide range of dysregulated gene expression patterns. It has been argued that understanding the heterogeneity of both DNA and RNA molecular profiles in a more integrative and quantitative manner is essential for advancing systematic approaches to alternative therapies and improving the accuracy of cancer prognosis and treatment prediction.
In this work, we present a novel representation of multi-omics profiles that is rich enough to account for the observed heterogeneity in cancer and facilitates the development of quantitative, integrated metrics of variation. Building on the network of molecular interactions provided by Reactome, we construct a library of “paired DNA-RNA aberrations.” These paired aberrations capture prototypical and recurrent patterns of dysregulation in cancer. Each pair, called a “Source-Target Pair” (STP), consists of a “source” regulatory gene and a “target” gene whose expression is likely controlled by the source gene. An STP is deemed “aberrant” if the source gene undergoes a DNA-level aberration (such as mutation, deletion, or duplication) and the target gene exhibits an RNA-level aberration, meaning its expression deviates from the normal range.
Given a set of M STPs, a sample profile can be classified into one of the 2^M possible configurations. We focus on subsets of STPs and corresponding reduced configurations, selecting tissue-specific minimal coverings. A minimal covering is the smallest group of STPs such that every sample in the population exhibits at least one aberrant STP from that group. These minimal coverings are computed using integer programming. Once a covering is defined, we can quantify cross-sample diversity by examining the variability of aberrant STPs across samples, which is measured using the entropy of the distribution over configurations.
We apply this methodology to data from the TCGA (The Cancer Genome Atlas) for six distinct tumor types: breast, prostate, lung, colon, liver, and kidney cancers. This approach allows us to efficiently simplify the complex molecular landscape observed across different cancer populations, uncovering novel signatures of molecular alterations that would not be detectable through traditional frequency-based methods. Our analysis reveals a stable pattern of cancer heterogeneity across tumor phenotypes: entropy increases as disease severity worsens.
This framework offers a powerful approach to understanding the intricate molecular landscape of cancer. It is well-suited to accommodate the growing complexity of cancer genomes and epigenomes as large consortia projects continue to expand, providing a valuable tool for refining cancer classification and improving therapeutic strategies based on molecular profiles.
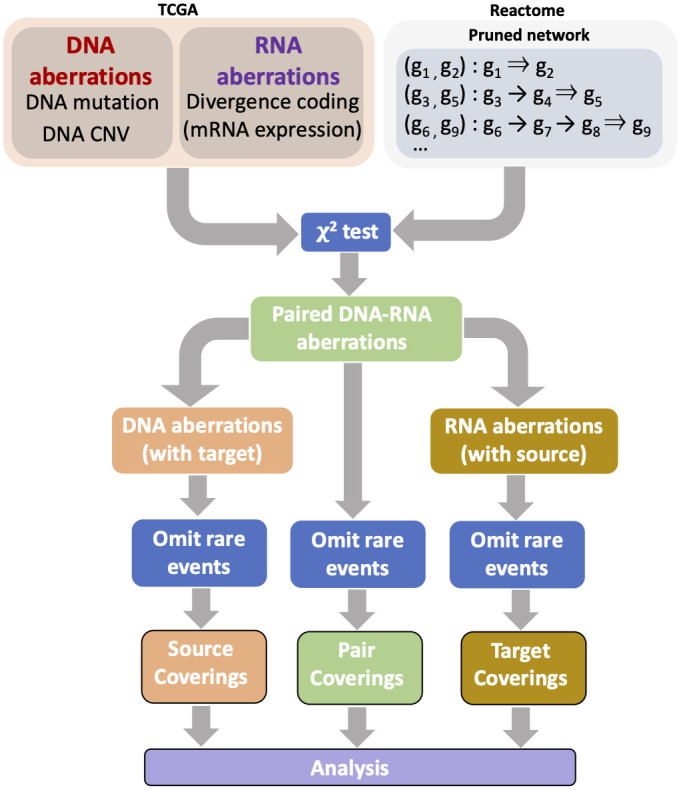